1.0 Introduction
The African trypanosomes responsible for sleeping sickness and nagana are cyclically transmitted by tsetse flies (Diptera: Glossinidae) [1]. The World Health Organization (WHO) estimate that there are approximately 50,000 deaths annually and a loss of 1,598,000 disability-adjusted life years (DALYs) caused by human African trypanosomiasis (HAT) with 60 million people at risk in 37 countries covering ∼40% of Africa (11 million km2) [2]. After a devastating epidemic in the early 20th century when a million people died of HAT, the disease nearly disappeared in the 1960s only to re-emerge strongly in the 1990s [3]. In addition, animal African trypanosomiasis or nagana has restricted agricultural development and human nutrition in sub-Saharan Africa and has a profound effect on the economy of much of the continent [4], as recognized by the African Union [5]. Despite the importance of these diseases, our understanding of tsetse/trypanosome interactions is still rudimentary [6].
Human African trypanosomiasis takes 2 forms, depending on the subspecies of the parasite involved: Trypanosoma brucei gambiense is found in 24 countries in west and central Africa [7]. This form currently accounts for 97% of reported cases of sleeping sickness and causes a chronic infection [8, 9]. A person can be infected for months or even years without major signs or symptoms of the disease. When more evident symptoms emerge, the patient is often already in an advanced disease stage where the central nervous system is affected [10].
Trypanosoma brucei rhodesiense is found in 13 countries in eastern and southern Africa. Nowadays, this form represents under 3% of reported cases and causes an acute infection [11]. First signs and symptoms are observed a few months or weeks after infection [12]. The disease develops rapidly and invades the central nervous system [13]. Another form of trypanosomiasis occurs mainly in Latin America. It is known as American trypanosomiasis or Chagas disease [14]. The causal organism belongs to a different Trypanosoma subgenus, is transmitted by a different vector and the disease characteristics are different than HAT [15].
High-throughput sequencing and microarray technology have been used to screen for differential gene expression in disease [16]. Gene sequencing technology can obtain the unknown genome sequence of individuals, and bioinformatics makes it possible to process this huge genome sequence information [17, 18].
In recent years, several studies have begun to use bioinformatics technology to search for biomarkers related to the incidence, diagnosis, and treatment of diseases from the genome sequence database of patients [19]. Although very few differential genes have been found to have therapeutic effects, bioinformatics methods also provide a new way for us to explore potential biomarkers of diseases [20, 21].
In this article, differentially expressed genes (DEGs) between trypanosome infected patient’s samples and normal samples were identified based on the data downloaded from Gene Expression Omnibus (GEO) database. Bioinformatics methods were used to construct a protein-protein interaction (PPI) network of DEGs. Meanwhile, function and pathway annotation of DEGs were performed based on Gene Ontology (GO) and Kyoto Encyclopedia of Genes and Genomes (KEGG) pathways databases. The present study hoped to provide a new view and evidence for the mechanism of trypanosome infection and identify new drug targets
- Materials and methods
2.1 Collection of microarray data of Trypanosome infected patients
The gene expression profile of GSE85996 was downloaded from the GEO database. It was established on the platform GPL6887 Illumina MouseWG-6 v2.0 expression bead chip (Illumina Inc., San Diego, CA, USA). The dataset contained 8 Trypanosome infected and 4 non-infected healthy controls.
2.2 Data preprocessing and identification of DEGs
The original expression datasets following background correction, normalization and probe summarization were converted into expression measures using the R Limma package The linear models for microarray data package in Bioconductor was used to identify DEGs according to the cut-off criteria: Adjusted P<0.00 and |log2 fold-change (FC)|>0.5 [22].
2.3 Gene ontology (GO) and KEGG pathway enrichment analysis of DEGs
GO is a widely used method for the unification of biology which collected structured, defined and controlled vocabulary for a large scale of genes annotation. Kyoto Encyclopedia of Genes and Genomes (KEGG) database is a collection of online databases regarding gene functions, enzymatic pathways and associates genomic information with higher-order functional information. To understand the biological functions and cellular pathways of the DEGs, the present study explores the Enrichr server [23] to identify GO categories and KEGG pathways according to the protocol described in previous studies [24, 25]
2.4 Analysis of protein-protein interaction (PPI) network and sub-networks Search Tool for the Retrieval of Interacting Genes (STRING; string-db.org) is a precomputed global resource designed to evaluate PPI information. In the present study, the STRING online tool [26 ] was used to analyze the PPI of DEGs and experimentally validated interactions with a combined score >0.4 were selected as significant.
3.0 Results
3.1 Identification of fifferentially expressed genes in Trypanosoma infected patients
A total of 20 differentially expressed genes including 3 upregulated genes (STAT1, FBXW17 and LRRC15), and 17 downregulated genes (Setbp1, Rxfp1, 5031414D18Rik, Dnm3os, Rxfp1, Serpine2, Rnase2a, Tnfaip8l3, Serpine2, Adap1, Nrg1, P2ry14, Vegfd, Aldh1a2, P2ry14, Fgfbp3 and Aldh1a2) in Trypanosoma infected patient (Table 1). The volcano plot visualized the differentially expressed genes based on statistical significance (-log10 P-value) versus magnitude of change (log2 fold change) and is useful for visualizing differentially expressed genes. The highlighted genes are significantly differentially expressed at a default adjusted p-value cutoff of 0.05 (red = upregulated, blue = downregulated) (Figure 1A). The plot density visualized the distribution of the values of the selected Samples (Figure 1B). The boxplot visualized the suitability of the data for differential expression analysis (Figure 1C), while the Uniform Manifold Approximation and Projection (UMAP) shows how the samples are related to each other. The number of nearest neighbors used in the calculation is indicated in the plot (Figure 1D).
Table 1: The list of differentially expressed gene associated with Trypanosome infection
ID | Gene. symbol | Gene title | log2(fold change) | -LOG10 (Pvalue) |
ILMN_2655721 | Stat1 | signal transducer and activator of transcription 1 | 1 | 4.701 |
ILMN_2615145 | Fbxw17 | F-box and WD-40 domain protein 17 | 0.726 | 4.984 |
ILMN_2756421 | Lrrc15 | leucine rich repeat containing 15 | 0.694 | 5.409 |
ILMN_2448997 | Setbp1 | SET binding protein 1 | -0.534 | 5.225 |
ILMN_1223585 | Rxfp1 | relaxin/insulin-like family peptide receptor 1 | -0.591 | 5.877 |
ILMN_2985657 | 5031414D18Rik | RIKEN cDNA 5031414D18 gene | -0.634 | 4.686 |
ILMN_1255731 | Dnm3os | dynamin 3, opposite strand | -0.659 | 5.638 |
ILMN_2685751 | Rxfp1 | relaxin/insulin-like family peptide receptor 1 | -0.709 | 4.706 |
ILMN_1246808 | Serpine2 | serine (or cysteine) peptidase inhibitor, clade E, member 2 | -0.757 | 4.949 |
ILMN_2890019 | Rnase2a | ribonuclease, RNase A family, 2A (liver, eosinophil-derived neurotoxin) | -0.797 | 4.897 |
ILMN_1245195 | Tnfaip8l3 | tumor necrosis factor, alpha-induced protein 8-like 3 | -0.872 | 5.018 |
ILMN_2883164 | Serpine2 | serine (or cysteine) peptidase inhibitor, clade E, member 2 | -0.945 | 5.483 |
ILMN_2538422 | Adap1 | ArfGAP with dual PH domains 1 | -0.974 | 5.789 |
ILMN_2971688 | Nrg1 | neuregulin 1 | -1.062 | 4.833 |
ILMN_3154419 | P2ry14 | purinergic receptor P2Y, G-protein coupled, 14 | -1.189 | 5.377 |
ILMN_2697220 | Vegfd | vascular endothelial growth factor D | -1.236 | 4.763 |
ILMN_2630753 | Aldh1a2 | aldehyde dehydrogenase family 1, subfamily A2 | -1.243 | 8.872 |
ILMN_1219200 | P2ry14 | purinergic receptor P2Y, G-protein coupled, 14 | -1.328 | 5.177 |
ILMN_2841593 | Fgfbp3 | fibroblast growth factor binding protein 3 | -1.355 | 4.764 |
ILMN_2630749 | Aldh1a2 | aldehyde dehydrogenase family 1, subfamily A2 | -1.728 | 7.602 |


3.3 Functional enrichment analysis of the differentially expressed genes The enriched KEGG pathway of the differentially expressed genes includes; the Relaxin signalling pathway, Retinol metabolism, ErbB signalling pathway, AGE-RAGE signalling pathway in diabetic complications, Neuroactive ligand-receptor interaction, TNF signalling pathway, Focal adhesion, Rap1 signalling pathway, Ras signalling pathway and Calcium signalling pathway (Figure 3). However, the enriched biological process of the differentially expressed genes included positive regulation of fibroblast growth factor receptor signalling pathway, cardiac endothelial cell differentiation, cardiac muscle cell myoblast differentiation, negative regulation of plasminogen activation, positive regulation of mast cell chemotaxis, endocardial cell differentiation, activation of transmembrane receptor protein tyrosine kinase activity, regulation of mast cell chemotaxis, regulation of striated muscle cell differentiation, and vitamin A metabolic process (Figure 4).
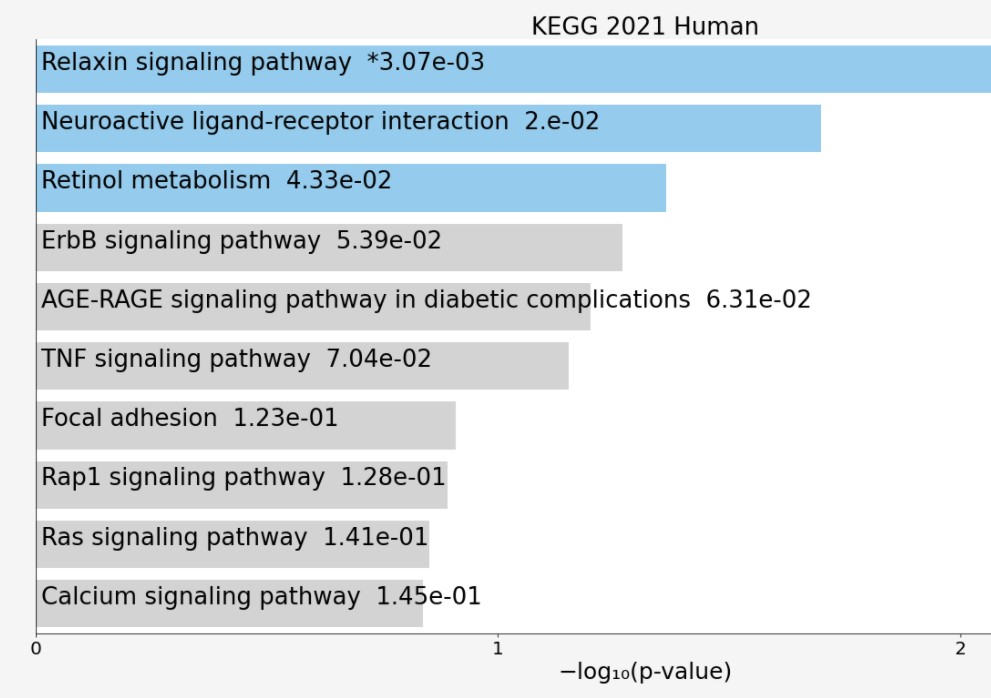
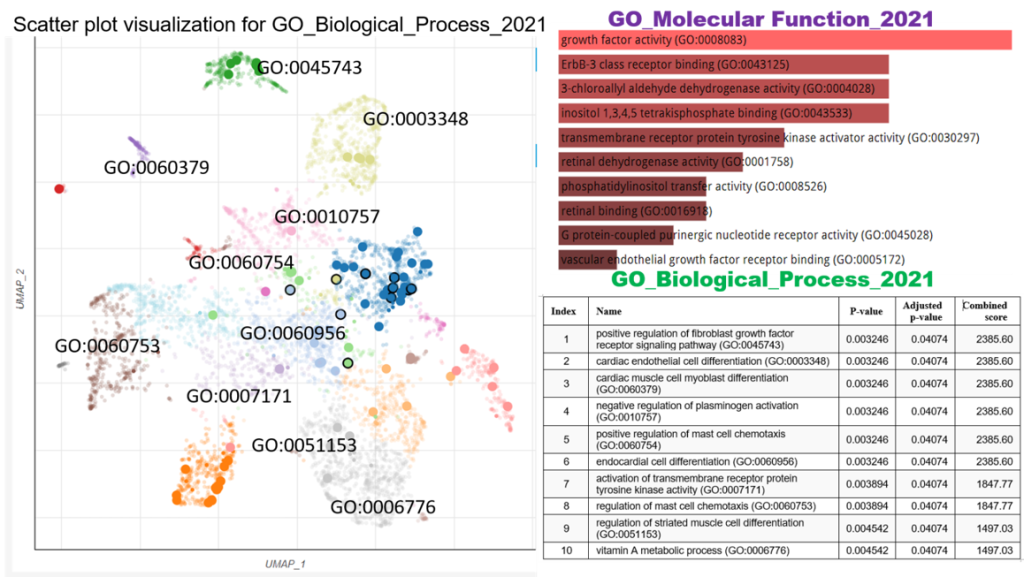
40 Discussion
The application and development of computer technology and mathematics in the field of biology (bioinformatics) has become one of the most important tools in proteomics [27]. Bioinformatics tools are essential for converting raw proteomics data into relevant knowledge and subsequently into useful applications [28]. Furthermore, bioinformatics provides a method to convert datasets into biologically interpretable results and functional outcomes. Many studies have successfully combined data mining with bioinformatics technology [29]. Through the analysis of these data, various key genes and signalling pathways related to trypanosome infection and pathogenesis were identified, which has resulted in a better understanding of the occurrence and development mechanism of the disease.
These bioinformatics analyses have shed light on the progression and pathology of trypanosome infection at the molecular level. We hypothesized that proteins that had been repeatedly identified by GEO proteomics studies may serve as potential biomarkers. However, the present study relies on bioinformatics mining of clinical data from patients. Therefore, differentially expressed genes or proteins that were reported in the articles must be experimentally validated.
We also used bioinformatics tools to carry out a GO (Gene Ontology) and KEGG (Kyoto Encyclopedia of Genes and Genomes) pathways analysis of DEG to observe and analyze the changes in proteins and signalling pathways during trypanosome occurrence and pathology from a global perspective.
GO analysis of the DEGs revealed that proteins related to the regulation of fibroblast growth factor receptor signalling pathway, cardiac endothelial cell differentiation, cardiac muscle cell myoblast differentiation, negative regulation of plasminogen activation, positive regulation of mast cell chemotaxis, endocardial cell differentiation, activation of transmembrane receptor protein tyrosine kinase activity, regulation of mast cell chemotaxis, regulation of striated muscle cell differentiation, and vitamin A metabolic process. This finding suggests that these biological processes may be closely associated with trypanosome infection and pathogenesis. The above GO pathways are known to promote the severity of trypanosome infection.
5.0 Conclusion
In conclusion, these hub genes identified in this study may have various roles in the occurrence, development, progression and severity of the trypanosomiasis, leading to damage of multiple systems in trypanosome infected patients. The present study may provide a basis for an improved understanding of trypanosome infection in humans. However, the current findings are limited by the lack of experimental verification in vivo and in vitro. Therefore, future experimental studies should be conducted to confirm the expression and function of the identified genes at the protein level, which may be an area of future research.
Funding
This research did not receive any specific grant from funding agencies in the public, commercial, or not-for profit sectors.
Author’s contributions
All authors contributed in preparing this article.
Conflict of interest
The authors declared no conflict of interest.
Acknowledgements
Not Applicable
References
1. Lehane, M.J.; Gibson, W.; Lehane, S.M. Differential expression of fat body genes in Glossina morsitans morsitans following infection with Trypanosoma brucei brucei. International Journal for Parasitology 2008, 38, 93-101, doi:https://doi.org/10.1016/j.ijpara.2007.06.004.
2. Organization, W.H. The world health report 2002: reducing risks, promoting healthy life; World Health Organization: 2002.
3. van Hove, D. Sleeping sickness in Zaire. The Lancet 1997, 349, 438.
4. Jordan, A.M. Trypanosomiasis control and African rural development; Longman: 1986.
5. Kabayo, J.P. Aiming to eliminate tsetse from Africa. Trends in Parasitology 2002, 18, 473-475.
6. Aksoy, S.; Gibson, W.C.; Lehane, M.J. Interactions between tsetse and trypanosomes with implications for the control of trypanosomiasis. 2003.
7. Brun, R.; Blum, J.; Chappuis, F.; Burri, C. Human african trypanosomiasis. The Lancet 2010, 375, 148-159.
8. Büscher, P.; Cecchi, G.; Jamonneau, V.; Priotto, G. Human african trypanosomiasis. The Lancet 2017, 390, 2397-2409.
9. Bashir, L.; Shittu, O.; Sani, S.; Busari, M.; Adeniyi, K. African natural products with potential antitrypanosoma properties: A review. Int J Biochem Res Rev 2015, 7, 45-79.
10. Jackson, A.P.; Sanders, M.; Berry, A.; McQuillan, J.; Aslett, M.A.; Quail, M.A.; Chukualim, B.; Capewell, P.; MacLeod, A.; Melville, S.E. The genome sequence of Trypanosoma brucei gambiense, causative agent of chronic human african trypanosomiasis. PLoS Neglected Tropical Diseases 2010, 4, e658.
11. Fevre, E.M.; Wissmann, B.v.; Welburn, S.C.; Lutumba, P. The burden of human African trypanosomiasis. PLoS neglected tropical diseases 2008, 2, e333.
12. Stich, A.; Abel, P.M.; Krishna, S. Human african trypanosomiasis. Bmj 2002, 325, 203-206.
13. Njiru, Z.K.; Mikosza, A.S.J.; Armstrong, T.; Enyaru, J.C.; Ndung’u, J.M.; Thompson, A.R.C. Loop-mediated isothermal amplification (LAMP) method for rapid detection of Trypanosoma brucei rhodesiense. PLoS neglected tropical diseases 2008, 2, e147.
14. Kirchhoff, L.V. American trypanosomiasis (Chagas’ disease)–a tropical disease now in the United States. N Engl J Med 1993, 329, 639-644, doi:10.1056/nejm199308263290909.
15. Schmuñis, G.A. Trypanosoma cruzi, the etiologic agent of Chagas’ disease: status in the blood supply in endemic and nonendemic countries. Transfusion 1991, 31, 547-557, doi:10.1046/j.1537-2995.1991.31691306255.x.
16. Wu, C.; Zhao, Y.; Lin, Y.; Yang, X.; Yan, M.; Min, Y.; Pan, Z.; Xia, S.; Shao, Q. Bioinformatics analysis of differentially expressed gene profiles associated with systemic lupus erythematosus. Molecular medicine reports 2018, 17, 3591-3598, doi:10.3892/mmr.2017.8293.
17. Lawal, B.; Kuo, Y.-C.; Tang, S.-L.; Liu, F.-C.; Wu, A.T.H.; Lin, H.-Y.; Huang, H.-S. Transcriptomic-Based Identification of the Immuno-Oncogenic Signature of Cholangiocarcinoma for HLC-018 Multi-Target Therapy Exploration. Cells 2021, 10, 2873.
18. Oshevire, D.B.; Mustapha, A.; Alozieuwa, B.U.; Badeggi, H.H.; Ismail, A.; Hassan, O.N.; Ugwunnaji, P.I.; Ibrahim, J.; Lawal, B.; Berinyu, E.B. In-silico investigation of curcumin drug-likeness, gene-targets and prognostic relevance of the targets in panels of human cancer cohorts. GSC Biological and Pharmaceutical Sciences 2021, 14, 037-047.
19. Li, N.; Qiu, L.; Zeng, C.; Fang, Z.; Chen, S.; Song, X.; Song, H.; Zhang, G. Bioinformatic analysis of differentially expressed genes and pathways in idiopathic pulmonary fibrosis. Annals of translational medicine 2021, 9, 1459-1459, doi:10.21037/atm-21-4224.
20. Wu, A.T.H.; Lawal, B.; Tzeng, Y.-M.; Shih, C.-C.; Shih, C.-M. Identification of a Novel Theranostic Signature of Metabolic and Immune-Inflammatory Dysregulation in Myocardial Infarction, and the Potential Therapeutic Properties of Ovatodiolide, a Diterpenoid Derivative. International Journal of Molecular Sciences 2022, 23, 1281, doi:10.3390/ijms23031281.
21. Wu, A.T.H.; Lawal, B.; Wei, L.; Wen, Y.-T.; Tzeng, D.T.W.; Lo, W.-C. Multiomics Identification of Potential Targets for Alzheimer Disease and Antrocin as a Therapeutic Candidate. Pharmaceutics 2021, 13, 1555.
22. Wu, S.-Y.; Lin, K.-C.; Lawal, B.; Wu, A.T.H.; Wu, C.-Z. MXD3 as an onco-immunological biomarker encompassing the tumor microenvironment, disease staging, prognoses, and therapeutic responses in multiple cancer types. Computational and Structural Biotechnology Journal 2021, 19, 4970-4983, doi:https://doi.org/10.1016/j.csbj.2021.08.047.
23. Chen, E.Y.; Tan, C.M.; Kou, Y.; Duan, Q.; Wang, Z.; Meirelles, G.V.; Clark, N.R.; Ma’ayan, A. Enrichr: interactive and collaborative HTML5 gene list enrichment analysis tool. BMC Bioinformatics 2013, 14, 128, doi:10.1186/1471-2105-14-128.
24. Khedkar, H.N.; Wang, Y.-C.; Yadav, V.K.; Srivastava, P.; Lawal, B.; Mokgautsi, N.; Sumitra, M.R.; Wu, A.T.H.; Huang, H.-S. In-Silico Evaluation of Genetic Alterations in Ovarian Carcinoma and Therapeutic Efficacy of NSC777201, as a Novel Multi-Target Agent for TTK, NEK2, and CDK1. International Journal of Molecular Sciences 2021, 22, 5895.
25. Mokgautsi, N.; Wang, Y.-C.; Lawal, B.; Khedkar, H.; Sumitra, M.R.; Wu, A.T.H.; Huang, H.-S. Network Pharmacological Analysis through a Bioinformatics Approach of Novel NSC765600 and NSC765691 Compounds as Potential Inhibitors of CCND1/CDK4/PLK1/CD44 in Cancer Types. Cancers 2021, 13, 2523.
26. Szklarczyk, D.; Gable, A.L.; Lyon, D.; Junge, A.; Wyder, S.; Huerta-Cepas, J.; Simonovic, M.; Doncheva, N.T.; Morris, J.H.; Bork, P., et al. STRING v11: protein-protein association networks with increased coverage, supporting functional discovery in genome-wide experimental datasets. Nucleic Acids Res 2019, 47, D607-d613, doi:10.1093/nar/gky1131.
27. Lawal, B.; Liu, Y.-L.; Mokgautsi, N.; Khedkar, H.; Sumitra, M.R.; Wu, A.T.H.; Huang, H.-S. Pharmacoinformatics and Preclinical Studies of NSC765690 and NSC765599, Potential STAT3/CDK2/4/6 Inhibitors with Antitumor Activities against NCI60 Human Tumor Cell Lines. Biomedicines 2021, 9, 92, doi:10.3390/biomedicines9010092.
28. Baxevanis, A.D.; Bader, G.D.; Wishart, D.S. Bioinformatics; John Wiley & Sons: 2020.
29. Chen, C.; Zhang, L.-G.; Liu, J.; Han, H.; Chen, N.; Yao, A.-L.; Kang, S.-S.; Gao, W.-X.; Shen, H.; Zhang, L.-J., et al. Bioinformatics analysis of differentially expressed proteins in prostate cancer based on proteomics data. OncoTargets and therapy 2016, 9, 1545-1557, doi:10.2147/OTT.S98807.