1.0 Introduction
The 2019 coronavirus disease also referred to as COVID-19 is caused by a new coronavirus termed Severe Acute Respiratory Syndrome Coronavirus-2 (SARS-CoV-2), which broke out in Wuhan city in December 2019 [1]. SARS-CoV-2 is the seventh member of the β-coronavirus family that afflict humans [2], and it is seemly more contagious and infectious than its close relatives, Middle East respiratory syndrome (MERS) and severe acute respiratory syndrome (SARS) [3]. SARS-CoV-2 is highly pathogenic, primarily targeting the human respiratory system thereby causing severe symptoms or even death [4, 5].
The virus has so far infected more than 400 million people with a death toll of over 5.7 million [6]. Considering the significant mortality and morbidity, as well as the social and economic burden posed by COVID-19 worldwide, it is therefore critical to continue the search for target specific chemotherapeutical agents to treat this viral disease. The main protease (Mpro) of SARS-CoV-2 plays a pivotal role in viral replication and transcription [7], and also in virus entry to host cells [5]. These functional properties of Mpro, coupled with the fact that no closely related homologues in human [7], makes it an attractive target for anti-viral drug development.
Plants are reservoirs of many bioactive molecules which have been traditionally explored in treating various human and animal diseases [8, 9]. Phytochemicals in plants such as flavonoids, polyphenols, terpenoids and alkaloids, play a crucial role in the drug discovery and development process [10]. Alkaloids in particular have been documented to possess a wide range of pharmacological activities, including antiviral, antioxidant, anti-inflammatory, antineoplastic and immunomodulatory properties [11, 12]. We recently profiled some herbal plants used in COVID-19 management in Southwestern Nigeria, which include Xylopia aethiopica (X. aethiopica) [13].
X. aethiopica, also known as Negro pepper, is an aromatic, evergreen tree native to the African Savanna region, specifically Ethiopia, Senegal, Ghana, Nigeria, and Cameroon [14]. The seed is widely used as a spice and in traditional medicine. It is a treasure trove of biological activities; it has been reported to possess anticancer, antidiabetic, antimalarial, analgesic, antioxidant, enzyme inhibitory, antimicrobial, and antibacterial and antiviral properties [14-16].
While isolation and characterization are critical steps in the identification of bioactive molecules in natural product drug discovery, computational techniques such as molecular docking, density functional theory (DFT), physiologically-based pharmacokinetic modelling, and others are becoming increasingly more popular for identifying of likely bioactive secondary metabolites in a timely and efficient manner [17]. For instance, the most widely used approach, DFT, has a lower computational cost than many other methods and is used to predict various properties of organic molecules including bond length, bond angles, dihedral angles and toxicity amongst others [18-20]. Molecular docking is commonly used to determine the appropriate orientation of molecules in the active site of the receptors of interest, and as well as determine their binding affinity. In essence, it is used to investigate the biological activity of molecules [21, 22].
Similarly, physiological-based drug metabolism and pharmacokinetic (DMPK) modelling that predict absorption, distribution, metabolism, excretion and the potential for toxicity (ADMET) in humans are critical to the success of any molecule. It is critical to implement some DMPK/ADMET-based limits early on in the discovery process in order to reduce the number of compounds required for evaluation and save time and resources [23]. Therefore, the bioactive compounds that can be isolated experimentally from medicinal plants can also be theoretically studied using these computational tools. Hence, given the rapid global spread of the coronavirus and the continual development of mutant strains that could lead to drug resistance, finding new anti-viral agents to reduce or end the epidemic health implications is critical. The primary objective is to evaluate potent pharmacological properties and the antiviral activity of presented oxoaporphine alkaloids against SARS-COV-2 main protease.
2.0 Methodology
2.1 Ligands and receptor selection
In this study, the oxoaporphine alkaloids previously identified in X. aethiopica were used [24]. These alkaloids were used as ligands against the Mpro target protein of SARS-CoV-2 retrieved from the protein data bank (https://www.rcsb.org/structure/6LU7). This three-dimensional crystal structure SARS-CoV-2 (PBD IDs: 6LU7) was selected because it was recently released, highly conserved in nature with high resolution (1.8 Å) and recognized by the X-ray diffraction technique. The three-dimensional structure of Mpro and its interactive co-crystallized ligand site on its structure is represented in Figure 1a.
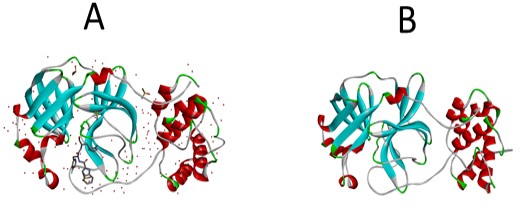
2.2 Receptor and ligands preparation
With the aid of discovery studio software, the inhibitor and water molecules were removed from the target protein (Figure 1b). This treated target protein was imported into Auto dock 4.2, where hydrogen atoms were added to the protein to correct the ionization and tautomeric states of the amino acid residues. Further, Kollman charges were added [25]. The dimensions of the grid box and receptor setup were x= 50 Å, y= 54 Å, z = 48 Å and x = -26.401 Å, y = 13.54 Å, z =40 Å during docking study, respectively, with a grid space of 1 Å. The accurate and reliable three-dimensional structure of the target protein was investigated using PROCHECK server which creates a Ramachandran plot, which shows the allowed and disallowed areas regarding backbone dihedrals of protein residues. Furthermore, the selected bioactive alkaloids from X. aethiopica (Figure 2, Table 1) were optimized using Spartan software. Optimized molecules were then saved in pdb format for molecular docking with the SARS-CoV-2 Mpro.
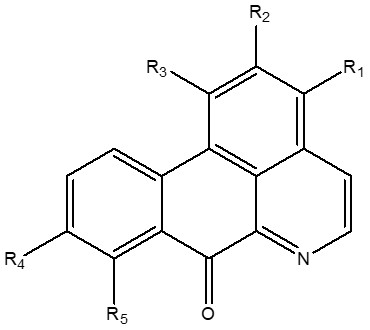
2.3 Pharmacokinetic study
The preADMET server (Korea) (https://preadmet.webservice.bmdrc.org/) and admetSAR server (http://lmmd.ecust.edu.cn/admetsar1) were used to predict the ADMET of all the studied alkaloids [26]. In this study, several parameters such as human intestinal absorption, Ames test blood-brain barrier, Caco-2 cell permeability, human Ether-à-go-go-Related Gene, carcinogenicity, molecular weight, hydrogen bond acceptor, hydrogen bond donor, topological polar surface area were considered.
Table 1: Substituent pattern on the oxoaporphine alkaloids in figure 2
Alkaloid | representation | R1 | R2 | R3 | R4 | R5 |
Liriodenine | Ligand 1 | H | O-CH2– | -O- | H | H |
Lysicamine | Ligand 2 | H | O-CH3 | O-CH3 | H | H |
o-Methylmoschatoline | Ligand 3 | O-CH3 | O-CH3 | O-CH3 | H | H |
Oxoglaucine | Ligand 4 | H | O-CH3 | O-CH3 | O-CH3 | O-CH3 |
Oxophoebine | Ligand 5 | O-CH3 | O-CH3 | O-CH3 | O-CH2 | -O |
2.4 Molecular docking of ligand-protein
To predict the binding affinity and activity of the ligand molecule, molecular docking is utilized to determine the scoring function and assess protein-ligand interactions [27]. The bioactive binding poses of the ligands dataset in the active site of SARS-CoV-2 Mpro were generated using the Auto dock tool. As a result, the scoring function was generated using the Lamarckian genetic algorithm’s usual technique [25]. During docking, target protein was centered on the grid map. The hydrophobic and non-bonded polar interactions at the inhibitor site of 6LU7 were modeled using Accelrys Discovery Studio 2019 software (Biovia, 2017). Discovery Studio Visualizer 4.0 was used to visualize the docking findings.
3.0 Results and discussion
3.1. Grid generation and protein structural reliability
The precise and dependable three-dimensional structure of the target protein is required for drug development. Figures 3 A and B shows that 91.2 percent of the residues in the target protein’s three-dimensional structure are in the most preferred area, while just 0.6 percent are in the prohibited zone (PDB ID: 6LU7). The requirement for becoming a model of excellent quality is to have more than 90% of residues in preferred areas.
3.2. Quantum Chemical Calculations.
Molecular chemical descriptors: highest occupied molecular orbital energy (EHOMO), lowest unoccupied molecular orbital energy (ELUMO), energy bandgap, log P, polarizability, dipole moment (DM), area volume, hydrogen bond donor, polar surface area and hydrogen bond acceptor (Table 3) were calculated for all the studied alkaloids via DFT with Becke three parameter exchange and Lee−Yang−Parr correlation (B3LYP) and 6-31+G(d,p) basis sets. The EHOMO, ELUMO and band gap energies provide qualitative information on the excitation characteristics and enzyme binding of modeled complex [28, 29] which in turn determines the extent of non-bonding interactions such as hydrophilic interactions and hydrogen bonding between the ligand and the receptor. The estimated EHOMO values, which reflect the potential of drug-like molecules to donate electrons to the nearby molecules, were −6.03 eV (liriodenine), −5.72 eV (lysicamine), −5.37 eV (o-methylmoschatoline), −5.54 eV (oxophoebine), and −5.24 eV (oxoglaucine). As shown in Table 3, oxoglaucine is expected to donate more electrons than the other oxoaporphine alkaloids investigated. The ELUMO demonstrate the compounds capability to accept electrons from neighboring molecules. Thus, the Liriodenine which has ELUMO of −2.40 eV, is predicted to have a better capability to accept electron from the neighboring molecule than other oxoaporphine alkaloids investigated. Band gap is an important parameter that indicates the extent of ligand-protein interaction between the bioactive compounds and the protein(s) under study. The lower the band gap, the better a compound’s ability to donate electrons to the neighbouring molecules. The order of the band gap is oxoglaucine < oxophoebine < o-methylmoschatoline < lysicamine < liriodenine (Table 3). Therefore, oxoglaucine is expected to react better than other studied compounds. The analysis of frontier molecule orbital density distributions of the compounds under study: HOMO (right), LUMO (left) (Table 4) shows that their electron density distribution on HOMO and LUMO are localized in the atoms of the aromatic cycles of the five compounds.
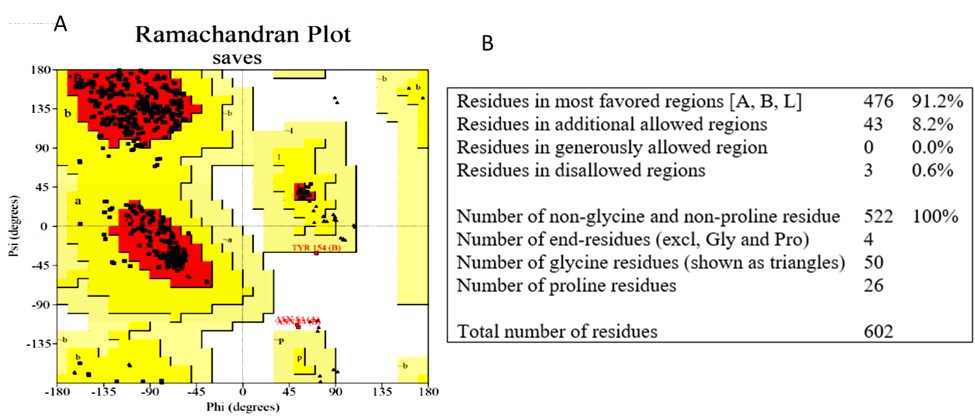
Table 3: Calculated molecular descriptors of the studied compounds
Alkaloids | EHOMO | ELUMO | BG | Log P | MW | DM | POL | HBD | HBA | OVA | PSA |
Liriodenine | -6.03 | -2.40 | 3.63 | 0.04 | 275.3 | 6.95 | 61.61 | 0 | 4 | 1.32 | 35.43 |
Lysicamine | -5.72 | -2.25 | 3.47 | 0.01 | 291.3 | 9.17 | 64.00 | 0 | 4 | 1.38 | 28.51 |
o-Methylmoschatoline | -5.37 | -2.13 | 3.24 | -0.97 | 321.3 | 9.38 | 66.25 | 0 | 5 | 1.42 | 30.51 |
Oxoglaucine | -5.24 | -2.09 | 3.15 | -0.95 | 351.4 | 10.68 | 68.49 | 0 | 6 | 1.48 | 39.84 |
Oxophoebine | -5.54 | -2.35 | 3.19 | -2.89 | 365.3 | 7.36 | 68.38 | 0 | 7 | 1.47 | 53.57 |
Notes:BG: band gap (ELUMO-EHOMO); DM: dipole moment; MW: molecular weight; OVA: ovality; POL: polarizability; HBD: hydrogen bond donor; HBA: hydrogen bond acceptor.
Table 4: Distribution of frontier molecule orbital density of studied compounds: HOMO (left), LUMO (right).
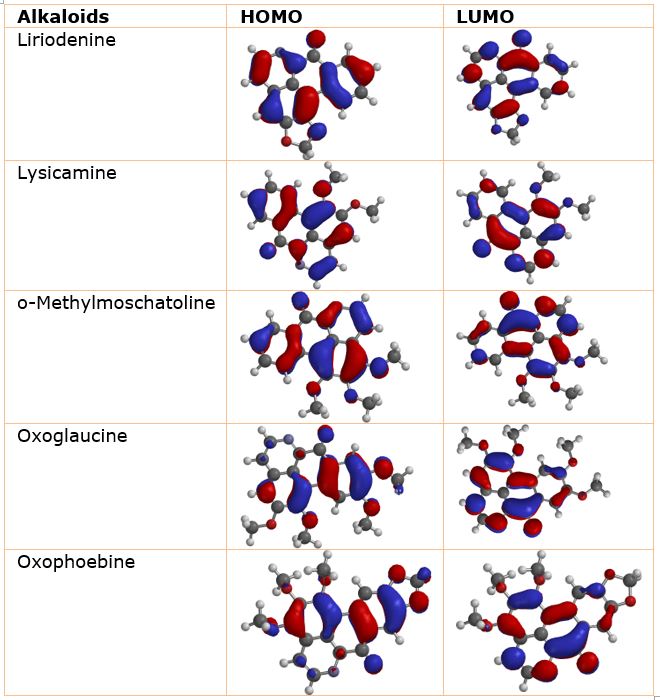
Lipophilicity is one of the essential physicochemical parameters for predicting the ‘drugability’ of molecules [30]. Lipophilicity refers to the ability of a molecule to partition into the lipid phase or biological membranes, and it can be measured or predicted experimentally by quantifying the distribution of a molecule between the aqueous and nonpolar phases [31, 32]. Lipophilicity, as the foremost drug-like parameter, has a significant impact on solubility, permeability, potency, selectivity, and other drug properties [32, 33]. Lipophilicity is commonly assessed using a calculated log P [34]. If the estimated log P is higher than the usual value for log P (log P ≤ 5), oral absorption, permeability and metabolic stability may be compromised, and the risk of promiscuity against metabolic enzymes and other hydrophobic targets other than the desired targets increases. When compared to Meanwell’s log P value [35], the calculated log P values (Table 3) are less than 5, indicating that the five oxoaporphine alkaloids were lipophilicity effective.
The dipole moment of a molecules is the product of the charge at both ends of the dipole of the molecule and the distance between the charges of molecules. The contribution of dipole moment to the biological activity of studied compounds is important. The presence of dipole moment as a non-bonded interaction in the drug-like compound-protein complex affect the relationship between ligand and the enzyme [36]. Furthermore, every single molecule investigated with higher DM value has been reported to have unique properties [37]. The DM of molecular compounds has been determined to range between 3 and 5 kJ/mol [38]. Practically, all of the DM values observed in this investigation are higher than the approved range.
3.3 Energetics and ligand-protein interactions
Docking studies were conducted to observe the interactions between the receptor and the ligand in order to determine the conformation of the molecules in the receptor gouge. It is also used to predict ligand-protein affinity [39]. Table 4 shows the binding affinity (kcal/mol) and interactions of various studied ligands orientations in the active site of the SARS-CoV-2 Mpro. Also, Figure 4 a-e, show the pictorial view of the docking simulations performed on the five oxoaporphine alkaloids.
The binding energies of the five alkaloids against the Mpro of SARS-CoV-2 vary from −7.0 to −7.9 kcal/mol, demonstrating that all the ligands had similar binding energies. However, the H-bond length between amino acids and the ligands spans from 1.88 to 3.68 kcal/mol (Table 5). It should be noted that the best ligand (a lead molecule) against COVID-19 is the molecule that binds to the SARS-CoV-2 Mpro with a desired effect. It is a compound with the highest binding energy expressed in terms of Gibbs free energy variation (ΔG) from thermodynamic standpoint [40, 41]. Apart from hydrogen bonding, which is the most powerful non-covalent contact for stabilizing complexes, there are a number of other non-covalent interactions that play a role [42], the five ligands show some similarities in interactions involving their aromatic rings. The presence of at least three aromatic rings in both compounds offers many possibilities for π-alkyl and π- π-stacking interactions to take place.
Table 5: Scoring of oxoaporphine alkaloids in X. aethiopica with SARS-CoV-2 Mpro (PDB ID: 6LU7)
Oxoaporphine Alkaloids | Scoring (kcal/mol) | Ki (μM) | Residues involved in interactions | Distance of H-bond between Amino Acid and ligand (Ӑ) | Types of Non-bonding interaction involved |
Liriodenine | -7.9 | 5.26 x 105 | HIS-41, CYS-44, MET-49, MET-165 | 2.71077, 3.66304 | Conventional hydrogen bond, Carbon hydrogen bond, Pi-Cation, Pi-Sulfur, Pi-Alkyl |
Lysicamine | -7.0 | 1.36 x 105 | GLU-166, CYS-145, MET-165, MET-49 | 2.16659, 3.61205 | Conventional hydrogen bond, Carbon hydrogen bond, Pi-Sulfur Pi-Alkyl, Van de Waals |
o-Methylmoschatoline | -7.0 | 1.36 x 105 | GLU-166, MET-49, CYS-44, CYS-145, HIS-41, | 3.48367, 3.43666 | Conventional hydrogen bond, Carbon hydrogen bond, Pi-Sulfur, Pi-Alkyl |
Oxoglaucine | -7.5 | 3.17 x 105 | GLU-166, MET-165, MET-49, LUE-141, CYS-145 | 2.17431, 3.59541 | Conventional hydrogen bond, Carbon hydrogen bond, Pi-Sulfur, Pi-Alkyl |
Oxophoebine | -7.6 | 3.75 x 105 | PHE-294, ASP-295, THR-111, | 1.8817, 3.68401 | Carbon hydrogen bond, Pi-Anion, Pi-Pi Stacked, Van de Waals |
Hydroxychloroquine sulfate | -6.1 | 2.97 x 104 |
Structurally, liriodenine (ligand 1) formed one H-bond with GLU-166, one carbon-hydrogen bond interaction with MET-49, π-alkyl interaction with CYS-145 and eight Van der Waals (vdW) interactions with HIS-41, CYS-44, TYR-54, PRO-52, ASP-187, GLN-189, GLN-192 and ARG-188.
Lysicamine (ligand 2) is characterized by one conventional H-bonding interaction with GLU-166, one carbon-hydrogen bond interaction with MET-49, four π-alkyl interaction: three with MET-165 and one with CYS-145, one π-sulphur with MET-165, eight vdW with HIS-41, CYS-44, TYR-54, MET-49, PRO-52, ASP-187, ARG-188, GLN-189 and GLN-192.
o-Methylmoschatoline (ligand 3) is characterized by zero conventional H-bonding interaction, two carbon-hydrogen bond interaction with THR-111, four π- π stacked with PHE-294, one π-anion with ASP-295, seven vdW with ASP-153, PHE-8, ASN151, THR-292, GLN-110 PRO-293 and ILE-249
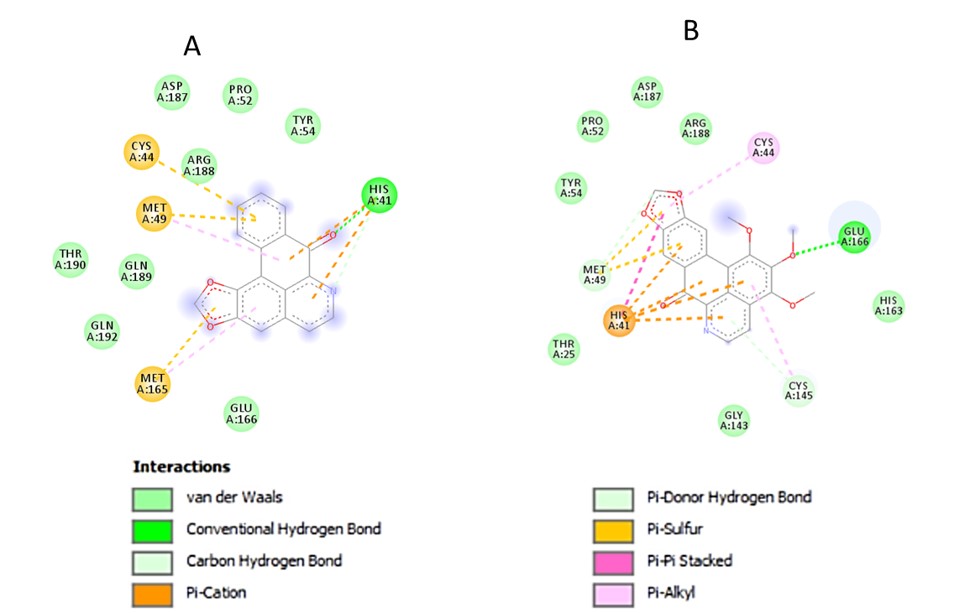
Oxoglaucine (ligand 4) stabilized its binding with the Mpro via one conventional H-bonding interaction with GLU-166, two carbon hydrogen bond interaction with MET-49 and LEU-141, four π- alkyl interaction with MET-165 and CYS-145, one π-sulphur with met 165, twelve vdW with GLY-143, ASN-142, SER-144, THR-25, HIS-41, CYS-44, TYR-54, PRO-52, ARG-188, ASP-187, GLN-189 AND GLN-192 (Figure 4)
Oxophoebine (ligand 5) is characterized by one conventional H-bonding interaction with GLU-166, one carbon hydrogen bond interaction with MET-49, four π-cation interaction with HIS-41, four π-alkyl interaction: two with MET-49, one with CYS-44 and one with CYS-145. Two π-sulphur with MET-49, five 165, twelve π- π stacked with HIS-41, π-donor hydrogen bond; Sulphur with CYS-145, ten vdW with GLN- 189, ARG-188, ASP-187, PRO-52, TYR-54, THR-25, GLY-143, ASN-142, HIS-163 and MET-165.
3.4. Prediction of physicochemical properties, drug likeness, pharmacokinetics and toxicity
An important parameter to consider during drug design is the physicochemical property of the molecules under consideration. The oral bioavailability of bioactive compounds were calculated through Lipinski’s rule of five and Veber’s rule [43], while the Muegge’s rule determined the possibility of a compound to become a successful drug molecule by the pharmacophore point calculation [44]. In Lipinski’s rule of five: molecular mass < 500; Hydrogen-bond donors (HBD) < 5; Hydrogen-bond acceptors (HBA) < 10; and Log P < 5 [45]. All the compounds adhered to the Lipinski’s, Veber’s and Muegge’s rule, and their values are shown in Table 6. Therefore, the five compounds were predicted to have good bioavailability and satisfied the drug likeliness parameters according to these rules. Consequently, the five oxoaporphine alkaloids may emerge as potential lead for SARS-CoV-2 Mpro.
The topological polar surface area (TPSA) gives the surface area containing polar atoms in a compound. When the TPSA is increased, a diminished membrane permeability is observed and compounds with higher TPSA were better substrates for p-glycoprotein, while ligands with lower TPSA value are better in penetrating to the central nervous system [46, 47]. Thus comparing the compounds TPSA, liriodenine, lysicamine and o-methylmoschatoline may be favorable than oxogluacine and oxopoebine (Table 6).
Table 6: Physiochemical properties and drug likeness of the five inhibitors
Physiochemical properties | Drug likeness | Lipophilicity | ||||||||
Ligands | MW | HBA | HBD | MR | TPSA | NRB | Lipinski violations | Veber violations | Muegge violations | MLog P |
1 | 275.26 | 4 | 0 | 76.7 | 48.42 | 0 | 0 | 0 | 0 | 1.71 |
2 | 291.3 | 4 | 0 | 83.6 | 48.42 | 2 | 0 | 0 | 0 | 1.54 |
3 | 321.33 | 5 | 0 | 90.1 | 57.65 | 3 | 0 | 0 | 0 | 1.21 |
4 | 351.35 | 6 | 0 | 96.6 | 66.88 | 4 | 0 | 0 | 0 | 0.9 |
5 | 365.34 | 7 | 0 | 96.2 | 76.11 | 3 | 0 | 0 | 0 | 0.77 |
MW=molecular weight, HBA=hydrogen bond acceptor, HBD= hydrogen bond donor, MR = molar refractivity, TPSA= topological polar surface area, NRB = number of rotatable bond.
Table 7: Pharmacokinetics and toxicity properties of the five inhibitors
Pharmacokinetic | Toxicity | |||||||
Ligands | GI absorption | HIA | CYP450 IP | BBB permeant | ESOL Class | Ames-test | Carcino-Rat/mouse | hERG |
1 | High | 0 | positive | Yes | Soluble | Mutagen | +/- | Medium risk |
2 | High | 0 | positive | Yes | Moderately soluble | Mutagen | +/- | Medium risk |
3 | High | 0 | positive | Yes | Moderately soluble | Mutagen | +/- | Medium risk |
4 | High | 0 | positive | Yes | Moderately soluble | Mutagen | +/- | Medium risk |
5 | High | 0 | positive | Yes | Moderately soluble | Mutagen | +/- | Medium risk |
GI = Gastrointestinal, HIA= Human Intestinal Absorption (HIA), IP = Inhibitory Promiscuity, BBB = Blood-Brain Barrier, hERG= human Ether-à-go-go-Related Gene, Carcino-= carcinogenicity.
The results of the pharmacokinetics and toxicity properties of the five oxoaporphine alkaloids are shown in Table 7. All five compounds show high human intestinal absorption. ADMET properties, as derived from the admetSAR server, reveal that the five studied compounds had better Human Intestinal Absorption (HIA) value than the hydroxychloroquine sulfate.
Figure 5b shows that ligands lysicamine, o-methylmoschatoline and oxoglaucine absorbed better than liriodenine and oxophoebine. Greater HIA denotes that the compound could be better absorbed from the intestinal tract upon oral administration. In the case of the hERG inhibition, all the ligands presented a medium risk with ligands 4 and 5 having low end point values of 0.5121 and 0.4838, respectively (Figure 5d).
Moreover, the rodent carcinogenicity in rat and mouse predicted by the preADMET server reveals that all are carcinogenic to rats and non-carcinogenic to mouse. The Ames test that assesses mutagenicity of a compound reveals that the five compounds are mutagenic. The probability of this mutagenicity (as depicted in figure 5c) shows that liriodenine and lysicamine have higher endpoint values. The blood-brain barrier (BBB) which selectively regulates the permeability of drugs to the brain shows that the penetration through the Blood-Brain Barrier (BBB) came out to be best for molecule liriodenine and oxophoebine but was not significantly higher than the hydroxychloroquine sulfate molecule (Figure 5a).
CYP450 inhibitory promiscuity property which is refers to the capacity for a drug or chemical to bind to and decrease or diminish the activity of multiple different CYP450 isoform enzymes.CYP450 inhibitory promiscuity values for all studied molecules are positive while that of hydroxychloroquine sulfate is negative.

4.0 Conclusion
In this study, quantum chemical method through DFT and docking methods were employed to verify the anti-protease activity of the oxoaporphine alkaloids of X. aethiopica. The docking study predicted steady conformations of the ligands in the active site of the enzyme and revealed that natural alkaloids: liriodenine, lysicamine, o-methylmoschatoline, oxoglaucine and oxophoebine had better binding free energies with Mpro of SARS-CoV-2 than hydroxychloroquine sulfate. The binding energy value for liriodenine indicates that it has a greater ability to inhibit SARS-CoV-2 Mpro than other alkaloids studied, which is further supported by the inhibition constant. Although toxicity properties of liriodenine, lysicamine, o-methylmoschatoline, oxoglaucine and oxophoebine are mutagenic, they have good oral availability and also meet RO5 criteria. Notwithstanding, there are literature evidences that structural modifications can mitigate their potential carcinogenic/mutagenic effects. These results are only preliminary screening to facilitate subsequent in vitro and in vivo investigations, and further structural-activity relationship in order to mitigate the predicted side effects.
Author contributions: OBT and OOO designed the project, OBT performed the in silico experiment. Both authors analysed the data and prepared the manuscript.
Conflict of interest: The authors declare no conflict of interest.
Funding: The authors did not receive any fund for the execution of this project.
References
1. Zhu, H.; Wei, L.; Niu, P., The novel coronavirus outbreak in Wuhan, China. Global Health Research and Policy 2020, 5, (1), 6.
2. Wu, D.; Wu, T.; Liu, Q.; Yang, Z., The SARS-CoV-2 outbreak: What we know. International Journal of Infectious Diseases 2020, 94, 44-48.
3. Zhang, Y.-y.; Li, B.-r.; Ning, B.-t., The Comparative Immunological Characteristics of SARS-CoV, MERS-CoV, and SARS-CoV-2 Coronavirus Infections. Frontiers in Immunology 2020, 11.
4. Ye, Z.-W.; Yuan, S.; Yuen, K.-S.; Fung, S.-Y.; Chan, C.-P.; Jin, D.-Y., Zoonotic origins of human coronaviruses. International journal of biological sciences 2020, 16, (10), 1686-1697.
5. Mengist, H. M.; Dilnessa, T.; Jin, T., Structural Basis of Potential Inhibitors Targeting SARS-CoV-2 Main Protease. Frontiers in Chemistry 2021, 9.
6. WHO World Health Organization Coronavirus (COVID-19) Dashboard. https://covid19.who.int/ (14-02-2022),
7. Jin, Z.; Du, X.; Xu, Y.; Deng, Y.; Liu, M.; Zhao, Y.; Zhang, B.; Li, X.; Zhang, L.; Peng, C.; Duan, Y.; Yu, J.; Wang, L.; Yang, K.; Liu, F.; Jiang, R.; Yang, X.; You, T.; Liu, X.; Yang, X.; Bai, F.; Liu, H.; Liu, X.; Guddat, L. W.; Xu, W.; Xiao, G.; Qin, C.; Shi, Z.; Jiang, H.; Rao, Z.; Yang, H., Structure of Mpro from SARS-CoV-2 and discovery of its inhibitors. Nature 2020, 582, (7811), 289-293.
8. Anand, U.; Jacobo-Herrera, N.; Altemimi, A.; Lakhssassi, N., A Comprehensive Review on Medicinal Plants as Antimicrobial Therapeutics: Potential Avenues of Biocompatible Drug Discovery. Metabolites 2019, 9, (11), 258.
9. Mushtaq, S.; Abbasi, B. H.; Uzair, B.; Abbasi, R., Natural products as reservoirs of novel therapeutic agents. EXCLI journal 2018, 17, 420-451.
10. Mgbeahuruike, E. E.; Yrjönen, T.; Vuorela, H.; Holm, Y., Bioactive compounds from medicinal plants: Focus on Piper species. South African Journal of Botany 2017, 112, 54-69.
11. Ghildiyal, R.; Prakash, V.; Chaudhary, V. K.; Gupta, V.; Gabrani, R., Phytochemicals as Antiviral Agents: Recent Updates. In Plant-derived Bioactives: Production, Properties and Therapeutic Applications, Swamy, M. K., Ed. Springer Singapore: Singapore, 2020; pp 279-295.
12. Ti, H.; Zhuang, Z.; Yu, Q.; Wang, S., Progress of Plant Medicine Derived Extracts and Alkaloids on Modulating Viral Infections and Inflammation. Drug design, development and therapy 2021, 15, 1385-1408.
13. Oderinlo, O. O.; Adenekan, O. A.; Alawode, T. T.; Osamudiamen, P. M.; Oluremi, B. B.; Oyeneyin, O. E.; Ngoepe, M. P., Ethnobotanical Appraisal and In-silico Investigation of Plants Used for the Management of COVID-19 in Southwestern Nigeria. 2021 2021, 7, (1), 24.
14. Yin, X.; Chávez León, M. A. S. C.; Osae, R.; Linus, L. O.; Qi, L.-W.; Alolga, R. N., Xylopia aethiopica Seeds from Two Countries in West Africa Exhibit Differences in Their Proteomes, Mineral Content and Bioactive Phytochemical Composition. Molecules (Basel, Switzerland) 2019, 24, (10), 1979.
15. Oluremi, B.; Adeniji, J., Anti-Viral Activity Evaluation of Selected Medicinal Plants of Nigeria Against Measles Virus. Microbiology Research Journal International 2015, 7, 218-225.
16. Tamfu, A. N.; Ceylan, O.; Kucukaydin, S.; Ozturk, M.; Duru, M. E.; Dinica, R. M., Antibiofilm and Enzyme Inhibitory Potentials of Two Annonaceous Food Spices, African Pepper (Xylopia aethiopica) and African Nutmeg (Monodora myristica). Foods 2020, 9, (12), 1768.
17. Kumar, M.; Singh, S. K.; Singh, P. P.; Singh, V. K.; Rai, A. C.; Srivastava, A. K.; Shukla, L.; Kesawat, M. S.; Kumar Jaiswal, A.; Chung, S.-M.; Kumar, A., Potential Anti-Mycobacterium tuberculosis Activity of Plant Secondary Metabolites: Insight with Molecular Docking Interactions. Antioxidants 2021, 10, (12), 1990.
18. Oyeneyin, O.; Adejoro, I. A.; Obadawo, B.; Amoko, J.; Olumide, I.; Akintemi, E.; Ipinloju, N., Investigation into the Molecular Properties of 3-(4-Hydroxyphenyl) Prop- 2-en-1-one 4-Phenyl Schiff Base and Some of Its Derivatives-DFT and Molecular Docking Studies. Science Letters 2021, 9, 4-11.
19. Townsend, P. A.; Grayson, M. N., Density Functional Theory in the Prediction of Mutagenicity: A Perspective. Chemical Research in Toxicology 2021, 34, (2), 179-188.
20. Hassan, T., Density Functional Theory Investigation of Some Pyridine Dicarboxylic Acids Derivatives as Corrosion Inhibitors. International Journal of Electrochemical Science 2020, 4274-4286.
21. Deghady, A. M.; Hussein, R. K.; Alhamzani, A. G.; Mera, A., Density Functional Theory and Molecular Docking Investigations of the Chemical and Antibacterial Activities for 1-(4-Hydroxyphenyl)-3-phenylprop-2-en-1-one. Molecules 2021, 26, (12), 3631.
22. Hussein, R. K.; Elkhair, H. M., Molecular docking identification for the efficacy of some zinc complexes with chloroquine and hydroxychloroquine against main protease of COVID-19. Journal of Molecular Structure 2021, 1231, 129979.
23. Sliwoski, G.; Kothiwale, S.; Meiler, J.; Lowe, E. W., Jr., Computational methods in drug discovery. Pharmacological reviews 2013, 66, (1), 334-395.
24. Fetse, J. P.; Kofie, W.; Adosraku, R., Ethnopharmacological Importance of Xylopia Aethiopica (DUNAL) A. RICH (Annonaceae) – A Review. Journal of Pharmaceutical Research International 2016, 11, 1-21.
25. Trott, O.; Olson, A. J., AutoDock Vina: improving the speed and accuracy of docking with a new scoring function, efficient optimization, and multithreading. Journal of computational chemistry 2010, 31, (2), 455-461.
26. Daina, A.; Michielin, O.; Zoete, V., SwissADME: a free web tool to evaluate pharmacokinetics, drug-likeness and medicinal chemistry friendliness of small molecules. Scientific Reports 2017, 7, (1), 42717.
27. Verdonk, M. L.; Cole, J. C.; Hartshorn, M. J.; Murray, C. W.; Taylor, R. D., Improved protein–ligand docking using GOLD. Proteins: Structure, Function, and Bioinformatics 2003, 52, (4), 609-623.
28. Bouachrine, M.; Hamidi, M.; Bouzzinea, S. M.; Taoufik, H., Theoretical study on the structure and electronic properties of new materials based on thiophene and oxadiazole. Journal of Applied Chemical Research 2009, 10, (2), -.
29. Semire, B.; Oyebamiji, A. K.; Ahmad, M. S., Theoretical Study on Structure and Electronic Properties of 2, 5-Bis [4-N, N- Diethylaminostyryl] Thiophene and Its Furan and Pyrrole Derivatives Using Density Functional Theory (Dft). Pakistan Journal of Chemistry 2012, 2, 166-173.
30. Arnott, J. A.; Planey, S. L., The influence of lipophilicity in drug discovery and design. Expert opinion on drug discovery 2012, 7, (10), 863-875.
31. Johnson, T. W.; Gallego, R. A.; Edwards, M. P., Lipophilic Efficiency as an Important Metric in Drug Design. Journal of Medicinal Chemistry 2018, 61, (15), 6401-6420.
32. Wang, J.; Skolnik, S., Recent Advances in Physicochemical and ADMET Profiling in Drug Discovery. Chemistry & Biodiversity 2009, 6, (11), 1887-1899.
33. Leeson, P. D.; Springthorpe, B., The influence of drug-like concepts on decision-making in medicinal chemistry. Nature Reviews Drug Discovery 2007, 6, (11), 881-890.
34. Arnott, J.; Kumar, R.; Lobo, S., Lipophilicity Indices for Drug Development. Journal of Applied Biopharmaceutics and Pharmacokinetics 2013, 1, 31-36.
35. Meanwell, N. A., Synopsis of Some Recent Tactical Application of Bioisosteres in Drug Design. Journal of Medicinal Chemistry 2011, 54, (8), 2529-2591.
36. Oyewole, R. O.; Oyebamiji, A. K.; Semire, B., Theoretical calculations of molecular descriptors for anticancer activities of 1, 2, 3-triazole-pyrimidine derivatives against gastric cancer cell line (MGC-803): DFT, QSAR and docking approaches. Heliyon 2020, 6, (5), e03926.
37. Debenedetti, P., Condensed matter. J of Phy 2003, 15, 1669.
38. Lewis, D. F. V.; Broughton, H. B., Molecular Binding Interactions: Their Estimation and Rationalization in QSARs in Terms of Theoretically Derived Parameters. The Scientific World Journal 2002, 2, 202353.
39. Gupta, M.; Sharma, R.; Kumar, A., Docking techniques in pharmacology: How much promising? Computational Biology and Chemistry 2018, 76, 210-217.
40. Mpiana, P. T.; Ngbolua, K.-t.-N.; Tshibangu, D. S. T.; Kilembe, J. T.; Gbolo, B. Z.; Mwanangombo, D. T.; Inkoto, C. L.; Lengbiye, E. M.; Mbadiko, C. M.; Matondo, A.; Bongo, G. N.; Tshilanda, D. D., Identification of potential inhibitors of SARS-CoV-2 main protease from Aloe vera compounds: A molecular docking study. Chemical Physics Letters 2020, 754, 137751.
41. Alfaro, M.; Alfaro, I.; Angel, C., Identification of potential inhibitors of SARS-CoV-2 papain-like protease from tropane alkaloids from Schizanthus porrigens: A molecular docking study. Chemical Physics Letters 2020, 761, 138068.
42. Enmozhi, S. K.; Raja, K.; Sebastine, I.; Joseph, J., Andrographolide as a potential inhibitor of SARS-CoV-2 main protease: an in silico approach. Journal of biomolecular structure & dynamics 2021, 39, (9), 3092-3098.
43. Veber, D. F.; Johnson, S. R.; Cheng, H.-Y.; Smith, B. R.; Ward, K. W.; Kopple, K. D., Molecular Properties That Influence the Oral Bioavailability of Drug Candidates. Journal of Medicinal Chemistry 2002, 45, (12), 2615-2623.
44. Muegge, I., Selection criteria for drug-like compounds. Medicinal Research Reviews 2003, 23, (3), 302-321.
45. Lipinski, C. A.; Lombardo, F.; Dominy, B. W.; Feeney, P. J., Experimental and computational approaches to estimate solubility and permeability in drug discovery and development settings. Advanced Drug Delivery Reviews 1997, 23, (1), 3-25.
46. Blake, J. F., Chemoinformatics – predicting the physicochemical properties of ‘drug-like’ molecules. Current Opinion in Biotechnology 2000, 11, (1), 104-107.
47. Chico, L. K.; Van Eldik, L. J.; Watterson, D. M., Targeting protein kinases in central nervous system disorders. Nature Reviews Drug Discovery 2009, 8, (11), 892-909.